Abstract
Late diagnosis of cancer and cardiovascular diseases often leads to poor chances of cure at high costs. An approach which has the potential to improve the status quo by helping to detect diseases early on, and thereby increase the chances of cure and reduce the costs for treatment, are longitudinal biomarker measurements of microRNA. In this report, we investigate the concept of a personalized baseline based on analysis of variance as well as hierarchical clustering for healthy/sick groups and individual patients in real data. Furthermore, we discuss mathematical models for the detection of illnesses from longitudinal miRNA data. For validation and verification of the proposed methods we develop a data augmentation strategy to generate a large volume of longitudinal miRNA data that can be used and continuously updated.
Content
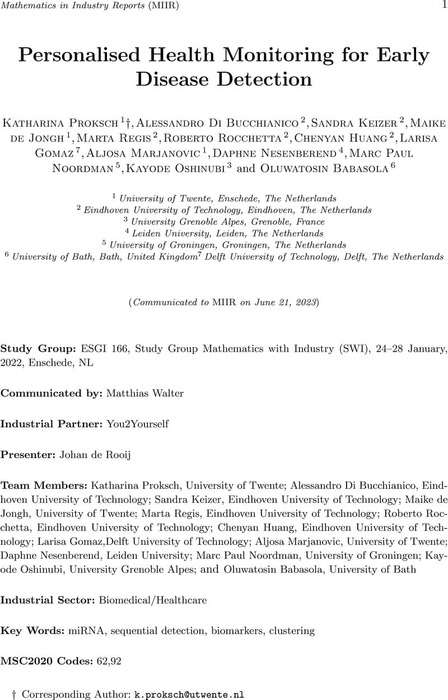