Abstract
Properly flying a walkalong glider is a challenging control problem, involving internal and external influences. We approach this problem from both a model-driven and data-driven viewpoint. We construct a mathematical model, based on well-established theory, which is used to derive both optimal paddle angle and distance between the paddle and the glider for steady level flight. Moreover, we derive parameters of the glider during uniform circular flight, as well as their relation to movement of the paddle from our model. As the glider is in practice very sensitive to airflow disturbances, we develope a data-driven model that tracks the status of the glider using video data, recorded by a camera mounted on the paddle. We use image processing and deep learning to automatically detect glider position with respect to the paddle and its yaw. Both approaches could be jointly used for feedback controlling an automatically operating walkalong glider.
Content
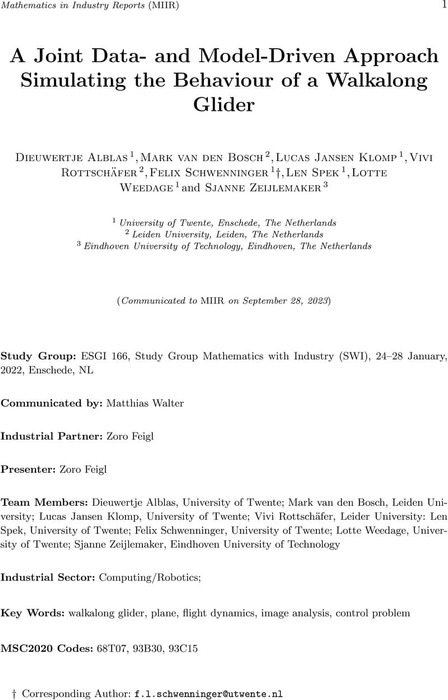