Abstract
Chronic Kidney Disease (CKD) is a progressive condition during which the kidneys fail to filter blood properly. Many moderate to late-stage symptoms exist for CKD but no prominent symptoms exist for early CKD, making it historically difficult to diagnose and treat. CKD can be triggered by infections, certain medications, dehydration, drug abuse, as well as spiraling blood pressure and blood sugar levels. Still, CKD is oftentimes overlooked when these triggers are present. For these combined reasons, we provide methodologies for cleaning pertinent data sets to conduct an exploratory analysis of patient profiles. Once we analyze how patient features correlate with CKD and identify important features for diagnosis, we train various machine learning classifiers to predict what stage of CKD a patient is in without invoking the features that are explicitly indicative of CKD. We then validate our models using out-of-sample test data.
Content
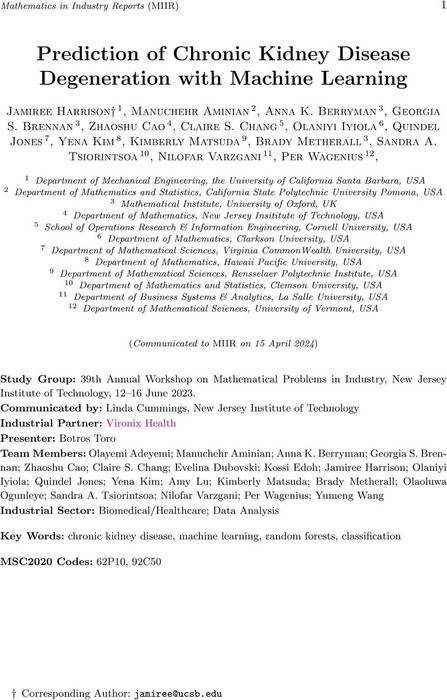